AI in Refineries: 5 Powerful Use Cases That Are Hard to Ignore
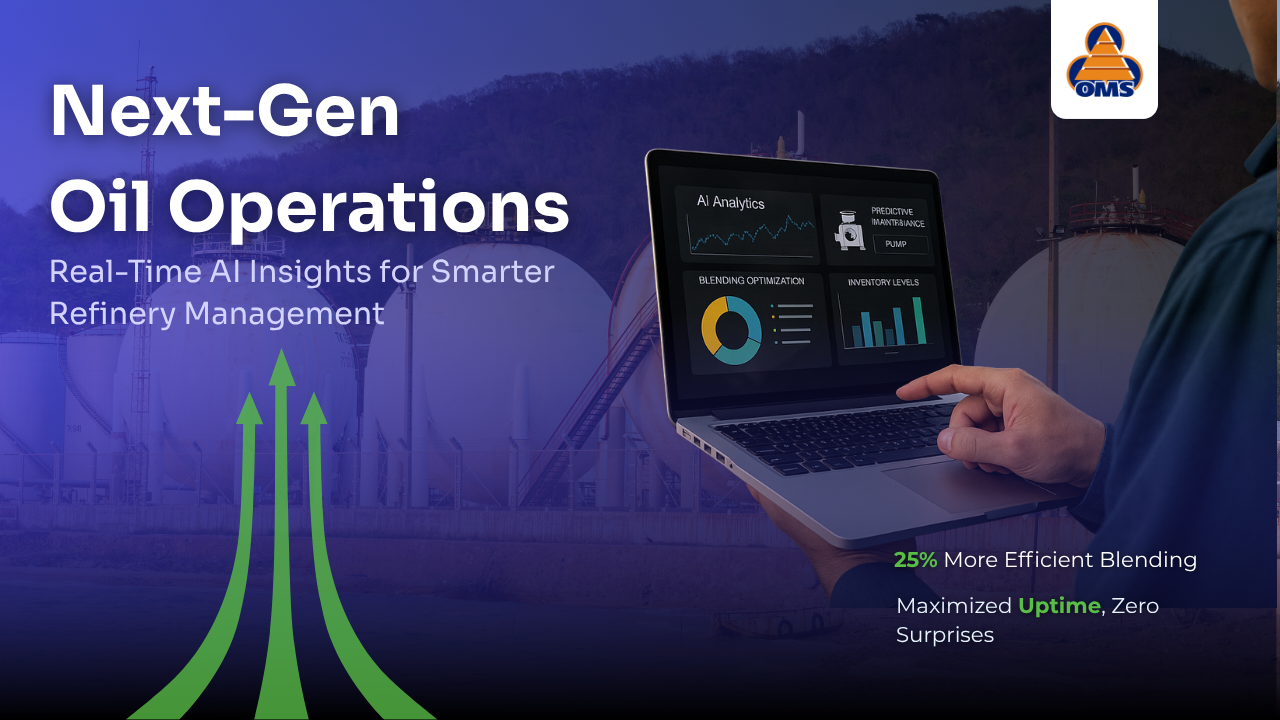
Table of Contents
Refineries’ offsite operations, blending, storage, and logistics are often overlooked, yet they heavily impact product quality, emissions, and efficiency. McKinsey estimates that AI can unlock $275 billion annually in the oil and gas industry by addressing off-site inefficiencies. Deloitte cites that oil companies often lose value by missing blend targets or circulating more than needed, but AI can help fix that by giving real-time insights to make better decisions.
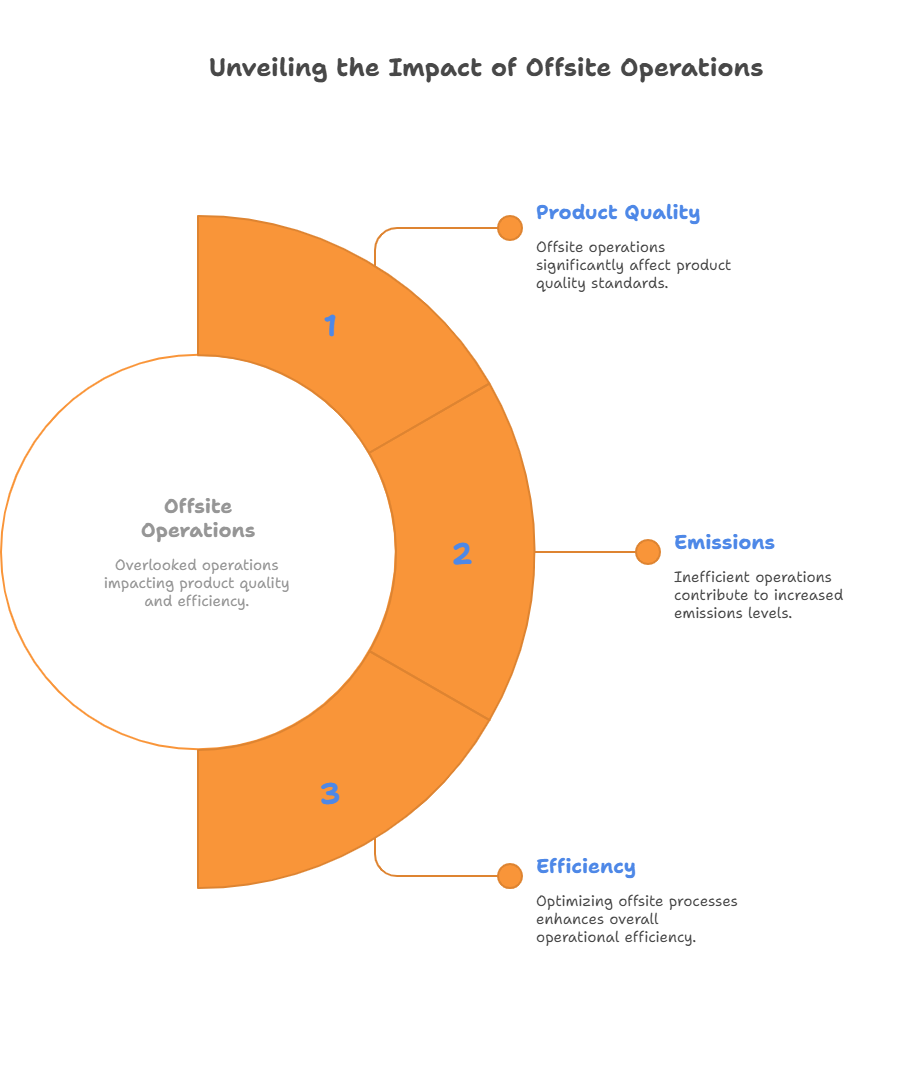
How AI-Driven Blending Is Transforming Refinery Operations
Blending is the last step before products leave the refinery, and it’s often where things go wrong. Traditional systems rely on rigid rules and offline analyzers, which means they react slowly and often fail to meet expectations. That leads to reblends, delays, and lost profits.
AI is changing the game. Tools like AspenTech’s Aspen MultiBlend utilize machine learning to adjust blend ratios in real-time, ensuring everything remains on spec while reducing giveaway and rework. AVEVA’s solutions go a step further by handling complex, multi-component blends with dynamic, on-the-fly optimization.
At OMS (Offsite Management Systems LLC), we take it even further with our hybrid First Principles + AI Blending Model (FPBM-AI). It combines deep engineering expertise with intelligent, adaptive capabilities. In a recent proof of concept (PoC) with Ecopetrol, it reduced reblends by 25% and increased margins.
This isn’t just automation—it’s a more innovative, more strategic way to run your blending operations in real time.
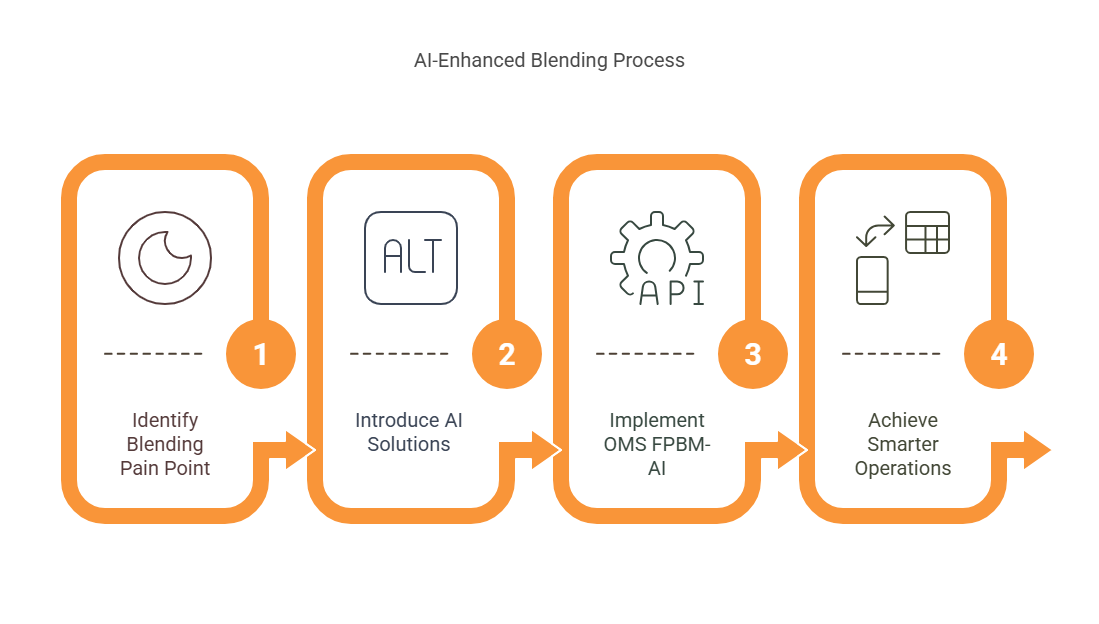
How AI Predictive Monitoring Prevents Equipment Failures in Refineries
In a refinery, pumps, valves, and movement control loops function like the circulatory system, ensuring operations flow smoothly. However, when one of these components fails without warning, it can cause costly delays or even bring entire processes to a halt.
Traditionally, maintenance has been either reactive (fix it after it breaks) or schedule-based (maintain it whether needed or not). Both approaches often result in either unnecessary upkeep or interventions that happen too late.
This is where AI changes the game. By converting real-time telemetry, like vibration signals, flow inconsistencies, or temperature anomalies, into actionable insights, AI systems learn to detect subtle signs of trouble long before failure occurs.
Leading platforms showcase this transformation:
- GE Digital’s Asset Performance Management (APM) leverages AI analytics to detect early warning signs hidden in sensor data. The result? Increased asset uptime and fewer unplanned shutdowns.
IBM’s Maximo APM offers real-time condition monitoring and predictive diagnostics for critical equipment like pumps, compressors, and valves. This enables maintenance teams to act early, minimizing disruption and extending asset life.
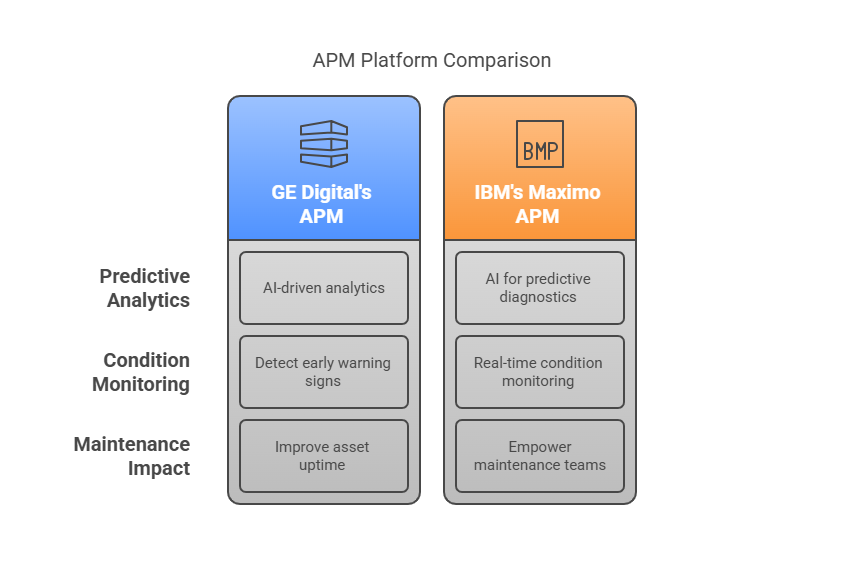
OMS’s AI-powered movement monitoring system detected early pump drift at a Middle East refinery—well before alarms triggered—enabling proactive maintenance and avoiding a $180,000 production loss. This predictive insight helps operators prevent downtime, ensuring movement systems run reliably, efficiently, and with no surprises.
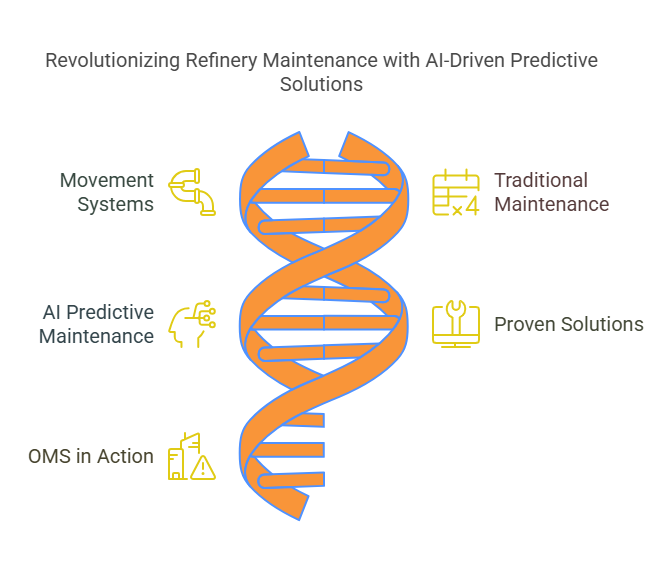
How AI Enhances Inventory Reconciliation and Loss Control in Refineries
Inventory reconciliation is critical for keeping refineries on track, ensuring mass balance, loss control, and regulatory compliance. However, it’s often disrupted by issues like sensor drift, manual entry errors, or unnoticed flow irregularities.
This is where AI makes a real difference. By analyzing data from tank levels, flow meters, and lab results, AI can reconcile volumes in real time, quickly flag inconsistencies, and catch problems before they escalate.
For instance, tools like Honeywell’s AI-powered tank systems and KBC’s Petro-SIM are already proving their value in the field. At one site in Southeast Asia, OMS applied its AI-based anomaly detection to correct a 3% volume error caused by a faulty radar, preventing a major reporting mistake and saving both time and money.
It’s a smarter, faster way to ensure accuracy and build trust with regulators and stakeholders.
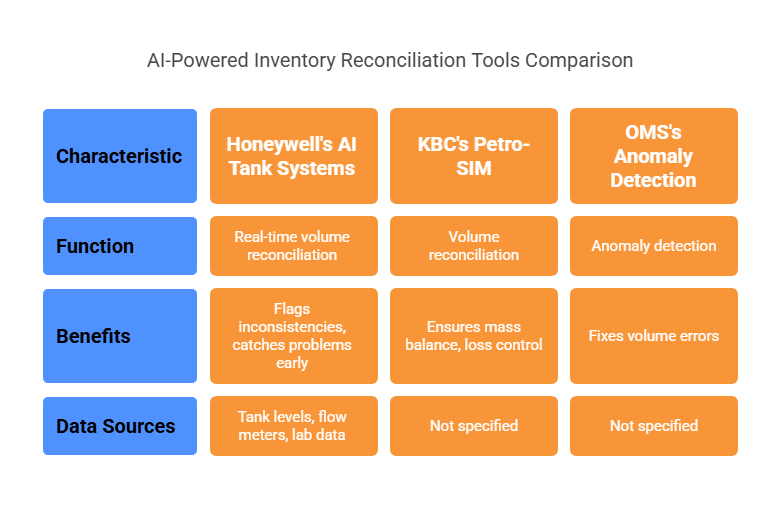
How AI-Powered Forecasting Improves Dispatch Scheduling in Refineries
Manual dispatch forecasting often depends on static assumptions and delayed signals, leading to overproduction, frequent tank switchovers, and tighter margins. This reactive approach struggles in today’s fast-changing market conditions.
AI introduces agility. By analyzing historical trends, real-time market signals, and operational limits, AI can predict demand more accurately and adaptively.
Technologies like ABB’s MPC-based scheduling systems and AVEVA’s Unified Supply Chain use machine learning to optimize dispatch in line with demand.
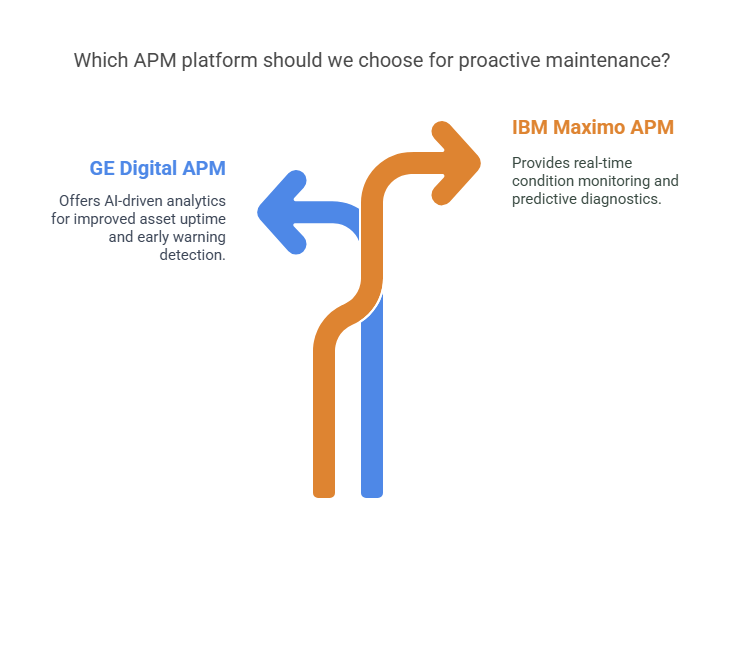
OMS enables intelligent scheduling through its AI-powered forecasting engine that anticipates market changes before they hit. At one major refinery, OMS predicted a diesel demand drop days before official data, allowing operators to delay blending and avoid producing 1,800 excess barrels.
This kind of foresight boosts agility, cuts waste, and sharpens commercial decisions.
The Hidden Challenges of Implementing AI in Brownfield Refineries
Even as AI proves its value across refinery offsite operations, deploying it in real-world brownfield environments presents its own set of hurdles. Legacy infrastructure, fragmented data, and cultural resistance often stand in the way of realizing AI’s full potential. Among these, data quality and integration remain the most foundational and frequently underestimated barriers.
Even as AI demonstrates its value across off-site refinery operations, real-world deployment—especially in brownfield environments—comes with unique challenges. Among these, data readiness is one of the most critical, yet often overlooked, barriers.
Key Challenges in Deploying AI in Brownfield Refinery Environments
- Legacy Infrastructure: Many refineries operate on decades-old systems that lack native AI compatibility.
- Fragmented and Siloed Data: Data exists across SCADA systems, PLCs, spreadsheets, and custom tools, making it hard to access and integrate.
- Data Quality Issues: Inconsistent formats, missing labels, and noise in historical data reduce its usefulness for training AI models.
- Cultural Resistance: Organizational inertia or fear of disrupting existing processes can slow down AI adoption.
Why Data Readiness Is Essential for Successful AI Deployment in Refineries
- AI thrives on clean, contextualized data. Without structured and reliable inputs, even the most advanced models produce unreliable results.
- Most refinery data is unstructured or isolated. SCADA systems and historians generate large volumes of data, but it’s often poorly labeled or difficult to access in real time.
- Bridging the gap starts with integration. Making this data usable requires a combination of cleaning, tagging, and synchronization with operational timelines.
Solutions in the Industry
- Platforms like Cognite’s DataOps demonstrate how industrial data, often noisy and unlabelled, can be contextualized, structured, and integrated to accelerate AI deployment.
- Likewise, Microsoft’s Energy Data Services provides a scalable architecture that layers structured access on top of SCADA and historian data, creating a unified view for AI applications.
Unlocking AI in refineries starts with AI-ready data. OMS’s historian-tap architecture extracts, cleans, and tags data non-intrusively, delivering structured, time-synced inputs for reliable ML insights. This enables legacy plants to adopt AI safely and efficiently—without disrupting control systems or requiring costly infrastructure changes.
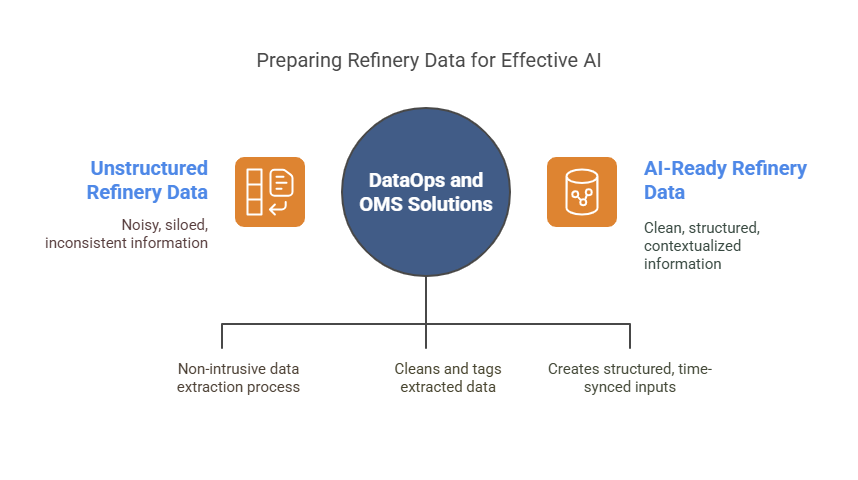
Conclusion
In today’s volatile energy landscape, refineries can no longer afford to overlook offsite operations. From blending and storage to logistics and forecasting, these areas significantly influence product quality, emissions, and profitability. AI offers a powerful way forward—enabling real-time insights, predictive monitoring, and smarter decision-making. By addressing challenges such as data fragmentation and legacy systems, refineries can unlock transformative value. OMS’s AI-driven solutions are designed to help refineries modernize without disruption, ensuring safer, more efficient, and resilient operations. As the industry evolves, embracing AI isn’t just strategic—it’s essential for long-term success and competitiveness in the global energy market.